
Data Pre-Processing
Two sets of seasonal LANDSAT TM and ETM+ spring and summer
images were obtained covering the period between 1985 and 2004.
Spring and summer images of the years 1985, 1987, 1991, 1997,
2001, 2003 and 2004 were obtained. The criteria of image
selection was based on the prevailing climatic conditions at
time of image acquisition and on the climate profile of the year
under investigation. Effort was made to select images from
years with similar climatic profiles (precipitation distribution
and temperature variation).
The satellite images were geometrically registered using a set
of ground Control Points (GCPs) collected using a differential
GPS. GPS points used in image registration were taken at
road intersections visible in the LANDSAT TM and ETM+ images.
Geometric registration accuracy of 1 pixel (30 meters) was
obtained in the registration process. Topographic relief
displacement was accounted for by orthorectification of the
LANDSAT TM Images by using the GCPs and and a Digital Terrain
Model (DTM). The modification in the polynomial equation
used with orthorectification as opposed to ordinary
rectification is that it takes into account terrain elevation,
local earth curvature, distance from nadir, and flying height
above datum to get a polynomial transformation between the image
and ground coordinates (ERDAS, 1997).
The second step in data pre-processing was the atmospheric
correction of the LANDSAT TM and ETM+ images. The
objective of an atmospheric correction is the elimination of
atmospheric effects so that the energy levels present on a
satellite image resemble those emitted by the features on the
earth’s surface so that changes in satellite image energy levels
can be attributed to changes in the surface and not to changes
in the atmosphere. The dark pixel subtraction method was
utilized to atmospherically correct the images. The
effectiveness The effectiveness of the method in reducing the in
between-scene illumination variation was quantitatively
resolved. This was accomplished by regressing the
surface-reflectance-corrected spectra of psuedo-invariant
targets between the three images for all bands. It was observed
that the regression coefficients are insignificantly different
from 1 which indicates that the psuedo-invariant targets have
the same reflectance values across the three images, therefore,
it can be concluded that the in between-scene illumination
variation was greatly reduced. However, the goodness of fit
values for the regression models, particularly for bands 1 and
2, were different from 1. This shows that the resultant
surface-reflectance-corrected spectra have residual artifacts.
This can be further corrected for by the calibration of remote
sensing data with in situ radiometric measurements made at the
same time of data acquisition. Although the removal of such
artifacts is necessary to extract biophysical information from
water bodies, most land-cover-related remote sensing
investigations do not require such high accuracy standards.
Top
Biomass Changes Detection
Following the atmospheric and geometric correction of the
satellite images, the images were analyzed. Several
spectral indices were derived that are indicators of green
biomass relative abundance but not measures of Biomass.
These were the Normalized Difference Vegetation Index (NDVI),
the Modified Soil Adjusted Vegetation Index II (MSAVI II) and
the Vegetation Reflectance Model (VR).
In order to measure biomass, the indicators of biomass relative
abundance were regressed against a set of field biomass
observations. This was achieved as follows:
-
First: fifty five plots were randomly selected in natural areas
occupied with herbaceous plant species. The plot
dimensions were approximately 90 by 90 meters.
-
Second: A number of quadrates ranging from 2 to 6 quadrates with
a dimensions ranging from 2 by 2 meters to 12 by 12 meters were
randomly selected within each plot. Green biomass within
each plot was harvested, oven dried and weighted.
-
Third: A Kriging spatial interpolation method between the
quadrates within each plot was used to derive a continuous
biomass surface for each plot.
-
Fourth: The plots were overlaid in a GIS over the derived
vegetation indices; namely NDVI, MSAVI II and VR model. The
vegetation indices were derived from the spring 2004 image
acquired over the same period of field observations.
-
Vegetation indices' values which are spatially corresponding to
plot locations were regressed against the measured Biomass
values (g/m2).
-
The regression equation was extended
spatially to calculate biomass from vegetation indices for the
entire study area and was after that extended temporally to the
other images to monitor changes from the year 1985 to the year
2004.
Figure 1 shows the regression results
between the 55 plots and the Normalized Difference Vegetation
index values obtained from the spring 2004 image. Regression coefficients were significant at the 99% confidence
level and the goodness of fit values for the regression model
was 0.77.
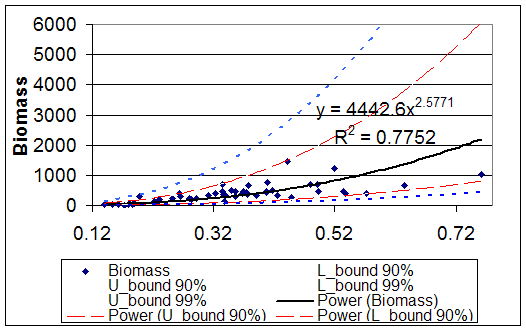
Figure 1. regression equation between NDVI values and
observed biomass (g/m2) for the training locations.
Top
Comparing the results from natural biomass analysis obtained from the multi-temporal set of satellite images has revealed a
significant drop in average natural biomass in the study area.
Figures 2a and 2b respectively show the drop in biomass as
observed in spring and summer seasons over the years from 1985
to 2004.
Figures 2.a and 2.b show the changes in average natural biomass
in gram per square meter for the study area.
Top
On the other hand, the measured biomass in agricultural areas
has slightly increased from the years 1985 to the year 2004
which indicates a more intensive cropping pattern. Figures
3a and 3b respectively show the increase in agricultural biomass
as observed in spring and summer seasons over the years from
1985 to 2004.
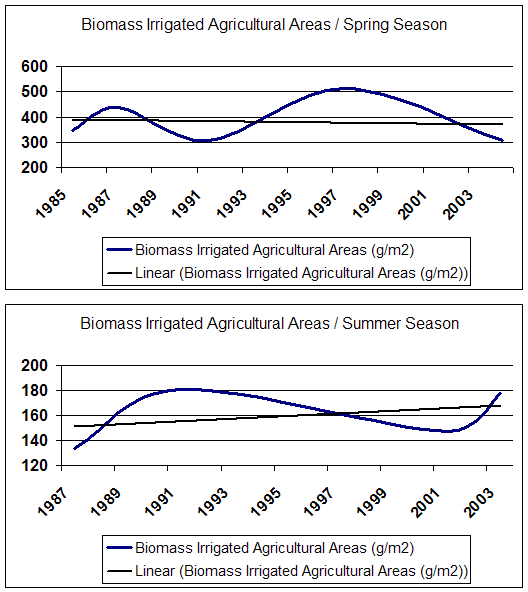
Figures 3a and 3b show the changes in average agricultural
biomass in gram per square meter for the study area.
Top
Land Use and Land Cover
Land use and
land cover are two approaches for describing land. Land use is
a description of the way that humans are utilizing any
particular piece of land for one or many purposes.
Comparatively, land cover is the bio-physical material covering
the earth's surface at any particular location. Together land
use and land cover information provide a good indication of the
landscape condition and processes that are occurring at a
particular place. Time series of land use/land cover maps tell
us how much of the landscape is changing, as well as what
changes have occurred and where the changes are taking place. Accurate and timely mapping of land use/land cover provides
vital information on the state of the environment, development
trends and wildlife habitat among others.
The land cover types identified
in the Dead Sea Basin were urban areas, road network, palm
trees, banana trees, olive trees, vineyards, citrus plantations,
other unclassified fruit trees, vegetables, wheat, natural trees
(forest), shrubs land, Natural grass land, open space with
little vegetation, open space with little or no vegetation, bare
soil & rocks and fallow agricultural land and water bodies.
Several sampling points were collected for each of the
aforementioned land cover types. The
spectral properties of the land cover types on the LANDSAT ETM+
image were inter-compared. The results can be summarized
as follows:
·
The three visible bands were not particularly
useful for the discrimination between mixed fruit trees, olive
grooves and banana plantations, these three classes had very low
reflectance values which overlap in the visible bands (Figure
3).
·
The near infrared (NIR) and the mid infrared (MIR)
were the best spectral regions for the discrimination between
vineyards and the other fruit tree classes found on the image.
The NIR and MIR values of vineyards were significantly higher
than the NIR and MIR reflectance values of other fruit trees;
however, there is still some overlap between vineyards
reflectance values in the NIR channel and the Palm trees
reflectance values (Figure 3). These findings were also verified
by the Euclidean-Distance separability index. The within land
cover averaged Euclidean Distance was similar to the between
land cover types Euclidean distance for the different fruit
trees classes (table 2).
·
The LANDSAT ETM+ reflectance bands did not provide
sufficient separability between all fruit trees classes except
for the vineyards class which is spectrally separable from the
other fruit trees.
·
Wheat and irrigated vegetables had similar
reflectance values in all bands.
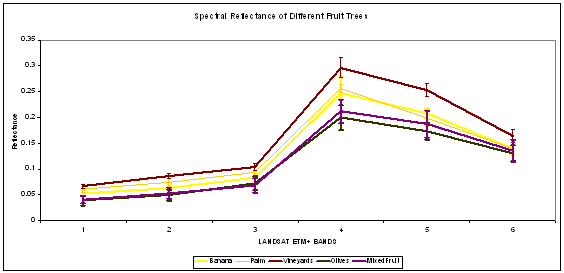
Figure 2: Spectral profiles of the different fruit tress types
found in the Dead Sea Basin
Top
Table 2: Averaged Euclidean Distance Separability Matrix the
different fruit tree types found in the Dead Sea Basin. In
yellow are the within group spectral variances. In orange
are the between groups (fruit tree types) spectral variance
with values similar to the within group spectral variances |
|
Wheat |
Vineyard JV |
Palm |
Banana |
Citrus |
Olives |
Fruit Trees |
Wheat |
0.1896177 |
0.2571188 |
0.2337757 |
0.2731029 |
0.2652369 |
0.2248768 |
0.2150513 |
Vineyard JV |
0.2571188 |
0.162351 |
0.1335741 |
0.1665519 |
0.1733696 |
0.1597859 |
0.1538814 |
Palm |
0.2337757 |
0.1335741 |
0.0682509 |
0.1445995 |
0.1324819 |
0.1312762 |
0.1320755 |
Banana |
0.2731029 |
0.1665519 |
0.1445995 |
0.1567811 |
0.1919255 |
0.1995885 |
0.1913182 |
Citrus |
0.2652369 |
0.1733696 |
0.1324819 |
0.1919255 |
0.1395421 |
0.185455 |
0.1902085 |
Olives |
0.2248768 |
0.1597859 |
0.1312762 |
0.1995885 |
0.185455 |
0.1154179 |
0.1146862 |
Fruit Trees |
0.2150513 |
0.1538814 |
0.1320755 |
0.1913182 |
0.1902085 |
0.1146862 |
0.1011312 |
An iterative process of refining the sampling areas in order to
maximize the between land cover types separability was
followed. This included deleting the sampling areas with high
within group variance values and adding new sampling areas with
low within group variance values.The procedure resulted in a
10% average increase in between groups’ Euclidean distance. Table 3 provides information as regards to the are of each land
cover class in the Dead Sea Basin.
Table 3
Areas of land cover types in the Dead Sea Basin |
CLASS NAME |
AREA SQ KM |
Banana
Grooves |
20 |
Citrus
Plantations |
2 |
Olive Trees |
67 |
Vineyard |
55 |
Palm Trees |
31 |
Fruit Trees
Unclassified |
129 |
Total fruit
Trees |
304 |
Fallow
Irrigated Ag Land |
97 |
Irrigated
Ag Land at time of Image Acquisition |
14 |
Wheat and
barely |
2 |
Total
Irrigated Ag Land |
113 |
Grass Land |
97 |
Natural
Shrubs |
49 |
Natural
Trees and Shrubs |
13 |
Open Space
With Little Vegetation |
865 |
Open Space
With Little or No Vegetation |
1,392 |
Bare Rocks |
630 |
Total
Natural and Semi-Natural Areas |
3,046 |
Water
Bodies |
886 |
Top
Accuracy assessment was
applied to evaluate the classification results and the overall
accuracy for the classification process.
An error matrix was determined and the Producer's (a measure of
omission error), User's Accuracy (a measure of commission error)
and the Kappa coefficient of agreement were calculated for each
class and for the overall map. The Kappa coefficient measures
the agreement between the classified and reference data
corrected for chance agreement (Congalton and Green, 1999). A
value greater than 0.80 represents strong agreement and a value
between 0.40 and 0.80 represents moderate agreement. A minimum
sample size of thirty points per class is generally recommended
for a valid accuracy assessment for that particular class.
Table 4 Accuracy Assessment results of the classification
procedure
Classified Data |
Reference |
Classified |
Number |
Producers |
Users |
|
Totals |
Totals |
Correct |
Accuracy |
Accuracy |
|
---------- |
---------- |
------- |
--------- |
----- |
Wheat & Vegetables |
32 |
27 |
18 |
56.25% |
66.67% |
Fruit Trees |
53 |
63 |
27 |
50.94% |
42.86% |
Forests and Shrubs |
38 |
22 |
15 |
39.47% |
68.18% |
Deep Water |
37 |
37 |
37 |
100.00% |
100.00% |
Shallow Water |
45 |
53 |
45 |
100.00% |
84.91% |
OSWLV |
67 |
77 |
64 |
95.52% |
83.12% |
OSWLNV |
41 |
40 |
40 |
97.56% |
100.00% |
Natural Grass L |
15 |
9 |
7 |
46.67% |
77.78% |
Bare Rocks |
22 |
22 |
22 |
100.00% |
100.00% |
Total |
350 |
350 |
275 |
78.57% |
|
Overall Classification Accuracy = |
78.57% |
|
|
|
Top |